If there is any single thing that I learned from my Biostatistics training that has served me best after all these years, it is the simple 2 x 2 table, often referred to as a contingency table. If there is one thing that I like to teach my students and residents, it is the utility of the 2 x 2 table in making sense out of data presented in journal articles. In fact, I consider it one of the most important tools (concepts) that you can master to further your understanding — and comprehension — of many of the concepts you will come across during your reading of clinical literature. Its beauty lies in its simplicity — and I like simplicity because it is easy to remember. Can you honestly tell me what the definition of sensitivity is? How about specificity? Do you get a little confused between these two important parameters involving a diagnostic test and an outcome or disease state? How about Positive Predictive Value (PPV)? While the definition ostensibly seems similar to sensitivity at a fast glance, it is critically different (and means something completely different as well). But the utility of these diagnostic parameters has great clinical significance when we rely upon a test to predict its ability to diagnose disease (or other outcome of interest). To keep things simple, I will not use any reference texts to explain this to you. As clinicians, we need to be able to lay out a 2 x 2 table in our head instinctively when we are presented data involving sensitivity, specificity, PPV, or negative predictive value. Furthermore, we must be able to use one of these parameters to determine another- even when we do not have the specific value presented to us. Again, it�s all in the Power of the Numbers!
RESIDENCY CRISIS in PODIATRY –
How Does This Affect You Personally? |
|
Currently, there is a shortage of residency training programs to meet the required demands of our graduates.
Interested and capable podiatrists in practice have many reasons to develop programs. Above the altruistic reasons there are direct personal benefits in starting residency training programs which may be significant. The following short video presentation will give you valuable insight and information. |
VIEW the video below for a BRIEF PRESENTATION by Edwin Wolf, DPM
SUMMARIZING the issue and where it currently stands |
|
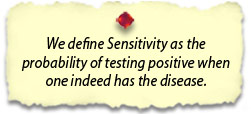 |
First, we need to recognize that for any given test for any disease or condition, there can be either a positive or a negative test result. If the test is positive when the disease is present (based on some other gold standard diagnostic test), it is termed a true positive test. If the test is negative in the presence of a person who really has the disease, this is a false negative test. Conversely, when a test result is negative in a person who does not have the disease, it is a true negative result. When the test result is positive in a person without disease, it is a false positive test result. While we count the crude numbers for each of these results, we generally refer to their frequencies as percentages within each group (i.e. percent of false positive results for the test). Sensitivity and specificity are similarly expressed as percentages (not rates) based on the crude numbers of test results as well. In this regard, we must always pay particular attention to the numerators and denominators (it�s all about numbers after all). We define Sensitivity as the probability of testing positive when one indeed has the disease. Think of it as the sensitivity of a test to detect the disease. The percentage (remember that probability is really a matter of percentages) is simply determined by dividing the number of true positive test results by the total number of persons with the disease (both true positives plus false negatives). Refer to my 2 x 2 contingency table in the figure. Here you can see the test results recorded in the table according to disease status.
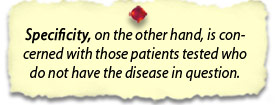 |
While you can lay out the contingency table any way that you like to place the data (test results by actual numbers) according to diagnosis, I prefer to lay it as you see because it makes sense to me. Here you can clearly see the two central columns indicating disease status (+ or -) and the two central rows indicating test results. Each cell therefore represents a specific type of
test result as previously referred to. For Sensitivity, we are concerned with those persons who truly have the disease (indicated in red text). Our formula for Sensitivity is simply (using numbers of results in each cell) : TP / TP + FN. The result is, of course expressed as a proportion. Specificity, on the other hand, is concerned with those patients tested who do not have the disease in question. A specific test is one that can reliably exclude persons without disease to a certain probability. Hence, in this regard we focus on those patients who have true negative test results (because they do not have the disease). Refer back to the 2 x 2 table again, but look at the column referring to patients who do not have the disease. The total number of non-diseased persons includes the number of false positive results plus the number of true negative test results (indicated in blue).
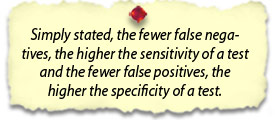 |
This total becomes our denominator for our specificity calculation, while the numerator is the number of true negatives. Specificity is therefore calculated by: TN / FP + TN. As you might imagine, based on these simple proportions, both sensitivity and specificity will be reduced by the percentage of false negative and false positive tests, respectively. Simply stated, the fewer false negatives, the higher the sensitivity of a test and the fewer false positives, the higher the specificity of a test. Since the total of true negative % plus false positive % must equal 100% , any test that has 20% false positive results must have a specificity of 80% (they are complements to each other). The same relationship exists between sensitivity and false negatives — easy to calculate one if you know the other.
Now what about Positive Predictive Value (PPV) of a test? This is defined as the probability of having the disease given a positive test result. In other words, how reliable is a positive test in diagnosing disease? In this regard, the denominator becomes the total number of positive tests (both true positive plus false positive). Referring back to our table, we are concerned with the horizontal row of positive test results. Since we are concerned with detecting those who actually have the disease, our number of true positives becomes the numerator once again. The calculation for PPV is therefore: TP / TP + FP. Again, as always, we have a percentage or proportion or probability. Again, the total probability must equal 1 (100%), so that if a test returns a 25% false positive result, we immediately know that the positive predictive value must be 75%. It�s simple math and proportions. You can determine these parameters easily, even when missing the results of one or two cells – just lay out the table and it is easy math as long as you have the totals.
|
See you next time.
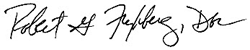
Robert Frykberg, DPM, MPH
PRESENT Editor,
Diabetic Limb Salvage
Suggested References:
- Statistics. Cliffs Notes, Lincoln Nebraska. David Voelker and Peter Orton. 1993
- Online Statistics Education: An Interactive Multimedia Course of Study (https://onlinestatbook.com/)
- Stanton Glantz. Primer of Biostatistics. McGraw – Hill, Inc., New York
- Theodore Colton. Statistics in Medicine. Little, Brown, and Company. Boston
Get a steady stream of all the NEW PRESENT Podiatry
eLearning by becoming our Facebook Fan.
Effective eLearning and a Colleague Network await you. |
|
This ezine was made possible through the support of our sponsors: |
Grand Sponsor |
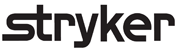 |
|
Diamond Sponsor |
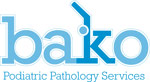 |
|
|
Major Sponsors |
|
|
|
|
|
|
|
|
|
|
|
|
|
|
|
|
|
|
|
|
|
|
|
|
|
|
|
|
|
|