In an effort to keep things simple, we will now focus on the very basic aspects of hypothesis testing and the meaning of that magical "statistically significant" result. When you read articles looking for important results between treatment groups or populations of patients, how do you know what differences between the populations are significant? Here is where the mystical "p-value" comes into play. I wonder how many of our readers actually know what a p-value represents? What does the "p" stand for? Well, it always comes down to probabilities. I have always remembered that the "p" stands for probability, percentage, or proportion, but the former is most accurate. I had a lot of trouble really understanding what the p-value meant other than that a significant value was represented by P<0.05. So what does this magical p<0.05 really mean that makes it statistically significant? Actually, it is not the number, but what the number represents. That is what always intrigued me about biostatistics – that we could make so much out of numbers. And remember, I am not a numbers or a math person!
When we test for significant differences between two (or more) groups, we are comparing differences between means, medians, survival, or percentages – depending of course what we are comparing. It is probably easier to compare the differences between the means of two populations. Let’s use age as the variable of interest in this regard. Say we have two populations of diabetic patients with Charcot foot, for instance. One of the groups (populations) is the group with type 1 diabetes with a mean age of 45 years. The second group is those with type 2 diabetes who also have Charcot foot syndrome. Their mean age is 53 years. If we have large enough groups such that their distributions are normal (see March issue for what this means), we can test the difference between the means using the infamous Student t-test for our sample populations. Our null hypothesis is of course that there is no difference between the two study groups and this is what we need to “reject” to determine a significant difference between the mean age of the two populations. While I do not want to confuse the issue at this point with the technicalities of hypothesis testing, it is easier to explain things in this fashion. Of course, numbers of individuals, statistical power, and standard deviation (spread) of the two population curves play an important part in this regard. Look at Figure 1. This represents (crudely) our two populations of normal distribution with age along the x-axis. We can see that there is a fairly good separation between the two population distributions with little overlap. It is precisely the overlap in the two populations that we are concerned with – the less the overlap, the greater the separation between means. The greater the separation, the greater the significance. To the contrary, when we see two groups that have little separation and that overlap quite a bit, we might also assume that both groups might actually come from the same population (have the same characteristics) but our sampling gives us slightly different means simply due to variability. We will come back to this example in the future when we formally discuss hypothesis testing, but for now, I just want to start you thinking about population distributions and parameters- and how they may or may not coincide depending on their characteristics.
Figure 1. This represents (crudely) our two populations
of normal distribution with age along the x-axis. |
|
|
|
To get back to our p-value, we might define it as the probability of obtaining a result as extreme or more extreme than the one computed when, in fact, there is really no difference between the two populations (i.e. they are really the same population). In other words, a p-value is the probability of being wrong when asserting that a true difference exists (i.e. when rejecting the null hypothesis). By convention, we are willing to accept a five percent (0.05) probability of being wrong when we state that there is, in fact a difference between study groups. When present, this is referred to as a Type 1 or α error and is customarily acknowledged as α=0.05.
In reading research papers, you want to look for p<0.05 to identify significant differences between groups since, although possible 5 percent of the time, there is little probability that the groups are the same. Obviously, the smaller the p-value, the lower the probability or possibility that the groups could truly be from the same population (Type 1 error). So when you see p<0.001, you can be fairly confident that the differences are robust and very significantly different.
P-values and population distributions become important also for determining 95% Confidence Intervals as well as for any type of statistical test you can think of. My goal is to make things easy to comprehend – in small doses so that you can assimilate these concepts as we move along. While I cannot give you in depth discussions or treatises on these subjects, I hope that my introduction to the basic concepts will help your understanding of these very important principles. As always, you should try to go to some of the sources I provide as references for more cogent discussions of individual subjects.
My hope is that you too will be amazed at the Power of Numbers...
See you next time.
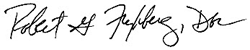
Robert Frykberg, DPM, MPH
PRESENT Editor,
Diabetic Limb Salvage
REFERENCES:
- Statistics. Cliffs Notes, Lincoln Nebraska. David Voelker and Peter Orton. 1993
- Online Statistics Education: An Interactive Multimedia Course of Study (https://onlinestatbook.com/)
- Stanton Glantz. Primer of Biostatistics. McGraw – Hill, Inc., New York
- Theodore Colton. Statistics in Medicine. Little, Brown, and Company. Boston
We at PRESENT love hearing from you. I would encourage you to share your experience, pearls, and wisdom on this topic, or on any other that you would like to share with our online community via eTalk.
Your continued participation is what makes this Web portal great!
Get a steady stream of all the NEW PRESENT Podiatry
eLearning by becoming our Facebook Fan.
Effective eLearning and a Colleague Network await you. |
|
Grand Sponsor |
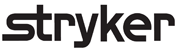 |
|
Diamond Sponsor |
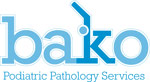 |
|
|
Major Sponsors |
|
|
|
|
|
|
|
|
|
|
|
|
|
|
|
|
|
|
|
|
|
|
|
|
|
|
|
|
|
|
|
|
|
|
|
|